ABSTRACT
Social Internet of Things (SIoT) has gained much interest among different research groups in recent times. As a key member of a smart city, the vehicular domain of SIoT (SIoV) is also undergoing steep development. In the SIoV, vehicles work as sensor-hub to capture surrounding information using the in-vehicle and Smartphone sensors and later publish them for the consumers. A cloud centric cyber-physical system better describes the SIoV model where physical sensing-actuation process affects the cloud based service sharing or computation in a feedback loop or vice versa.
The cyber based social relationship abstraction enables distributed, easily navigable and scalable peer-to-peer communication among the SIoV subsystems. These cyber-physical interactions involve a huge amount of data and it is difficult to form a real instance of the system to test the feasibility of SIoV applications. In this paper, we propose an analytical model to measure the workloads of various subsystems involved in the SIoV process. We present the basic model which is further extended to incorporate complex scenarios. We provide extensive simulation results for different parameter settings of the SIoV system. The findings of the analyses are further used to design example adaptation strategies for the SIoV subsystems which would foster deployment of intelligent transport systems.
RELATED WORKS
Vehicular communication technology is growing rapidly. A large body of researchers are working on the design and development of the communication standards and related routing protocols. Asthe communication technology is maturing, we witness research works on information dissemination over the vehicular networks. Consequently, SIoV is emerging as a promising application of big data from the IoT philosophy where vehicular sensor data will be consumed by different types of clients through the social network of services. There are few works related to VANETs traffic or safety message dissemination modeling from communication and routing perspective. In this paper, we propose application centric workload models of SIoV subsystems which will be helpful to build SOA, cloud computing or social network based IoV applications.
SOCIAL INTERNET OF VEHICLES
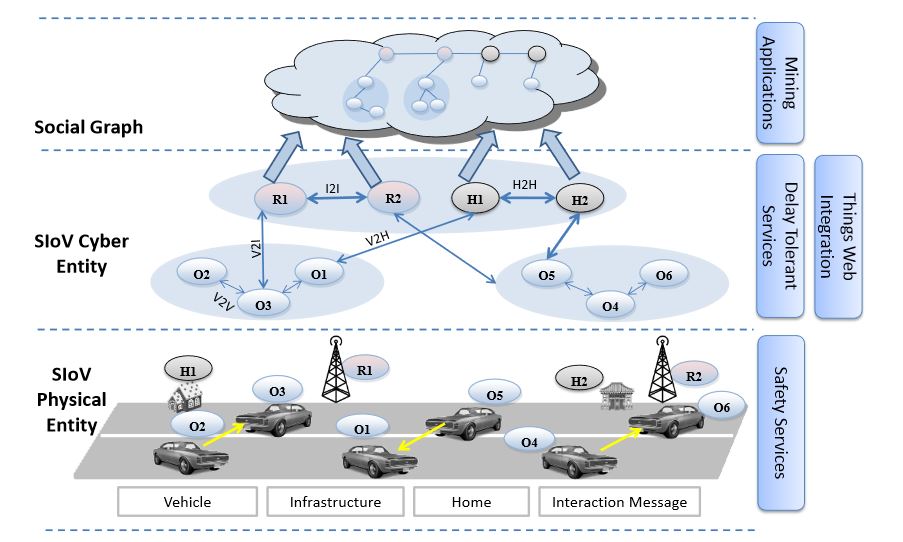
Figure 1. Architecture of Social Internet of Vehicles
The Social Internet of Vehicles (SIoV) (Figure 1) is a type of cloud based cyber-physical system where physical systems involved in VANETs have virtual entities, similar to the virtual sensors of global sensor network. This middleware based cyber or virtual computing maintains one-to-one feedback loop with the physical processes. Service computing or sharing affects physical sensing or actuation and vice versa. The cloud based cyber entities enable virtual peer-to-peer networking which fosters heavily decentralized cloud operation (e.g., fog computing). SIoV uses cyber-physical abstraction to form machine-to-machine (M2M) social network in the IoV domain to offer services related to safety, efficiency, comfort and entertainment applications.
THE BASIC MODEL
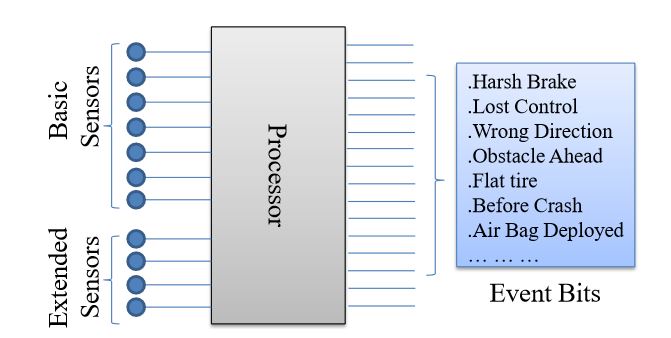
Figure 3. Sensory data to events identification
We assume that the vehicle is travelling at a constant speed (v) in between the infrastructures that are L distance apart, there is no traffic jam, the traffic is one way and each vehicle has rv communication range. Every vehicle is equipped with basic and extended sensors. Basic sensors are used to generate public information for safe manoeuvring (e.g., VANET “i am here” message). On the other hand, extended sensors generate intermittent messages that describe events occurring in and around the vehicle (e.g., sharp brake, lost control, obstacles ahead, wrong direction, etc.) (Figure 3). In addition, messages also contain the interaction information (e.g., efficiency, comfort, entertainment requests) which is different from the requested content.
We assume that the vehicle is travelling at a constant speed (v) in between the infrastructures that are L distance apart, there is no traffic jam, the traffic is one way and each vehicle has rv communication range. Every vehicle is equipped with basic and extended sensors. Basic sensors are used to generate public information for safe manoeuvring (e.g., VANET “i am here” message). On the other hand, extended sensors generate intermittent messages that describe events occurring in and around the vehicle (e.g., sharp brake, lost control, obstacles ahead, wrong direction, etc.) (Figure 3). In addition, messages also contain the interaction information (e.g., efficiency, comfort, entertainment requests) which is different from the requested content.
EXTENSION OF THE BASIC MODEL
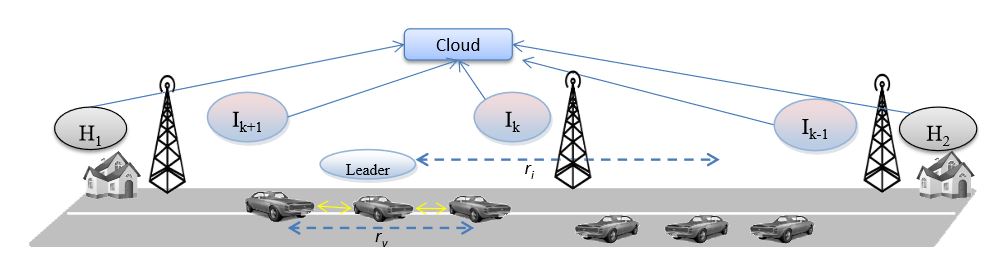
Figure 6. A scenario of the extended model
The basic model describes the SIoV scenario where one vehicle belongs to a household and travels from one infrastructure to the next infrastructure. We further extend the basic model to, (1) incorporate more vehicles to the dynamic scenario so that the vehicles can form platoons of multiple members; (2) introduce a region with multiple infrastructures so that inter-infrastructure communication can be logged; (3) add more houses to the residential areas to extend the number of local and remote friends (Figure 6). Following sections describe the models in detail.
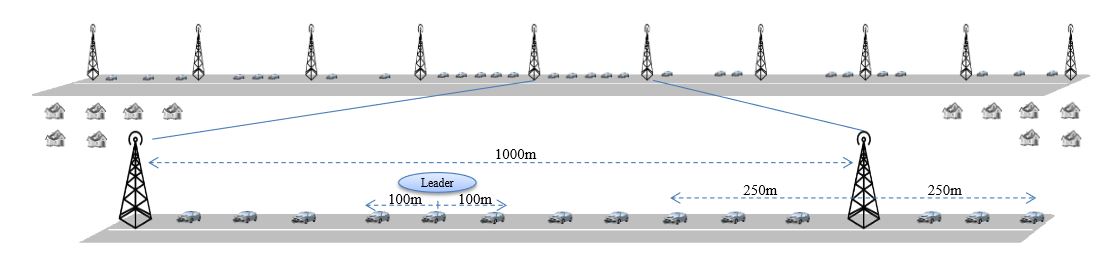
Figure 8. A detailed scenario setup of the extended model
After introducing more infrastructures to the last scenario, the existing model gets extended to a region of infrastructures (Figure 8). In this case, we have more V2I and I2I interactions in addition to more V2V interactions. In case of V2I interactions, once a vehicle reaches the range of an infrastructure, it uses push method to share the OBU-OBU social graph. We assume the number of vehicles approaching an infrastructure in the one-way scenario per unit of time (e.g., a second) is a Poisson process.
SIOV CHARACTERISTICS ANALYSIS
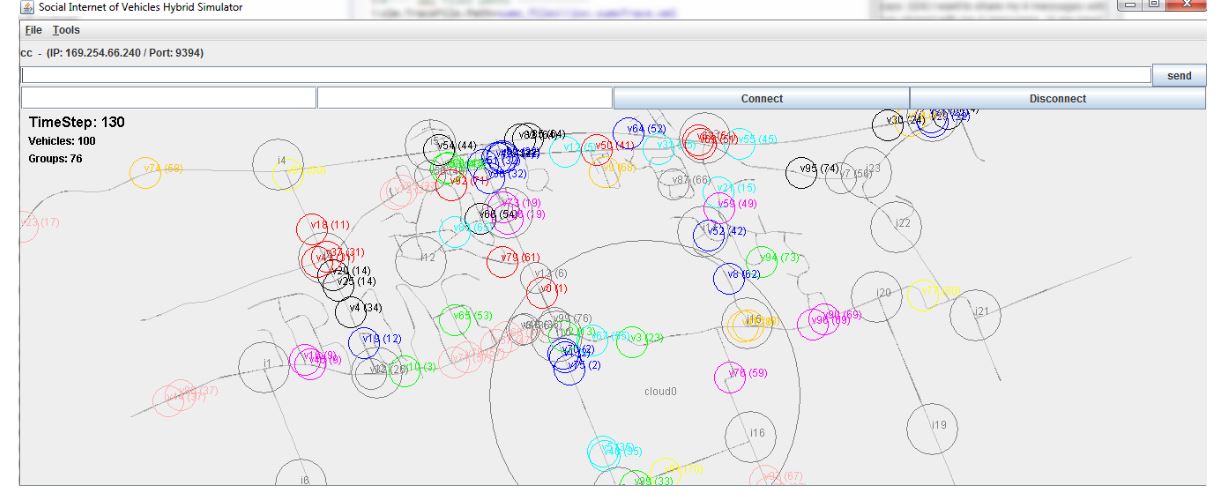
Figure 10. In this simulation window, we present 100 simulated vehicles, 25 infrastructures and 1 social graph cloud on the Cumberland, Ottawa, Canada region
We have designed and developed a simulator (Figure 10) that uses Open StreetMap (https://www. openstreetmap.org) for map area selection, SUMO to generate mobility traces on the map, JAVA to generate virtual sub-system processes and sockets for peer-to-peer network communication. The map data can further be augmented with sub-system (i.e., infrastructure, home, cloud) details. For the simulation measurement, at first medium scale vehicle mobility traces are generated using SUMO which are further customized by changing the simulator parameters such as vehicle arrival rate (ρ), and vehicle speed (v).
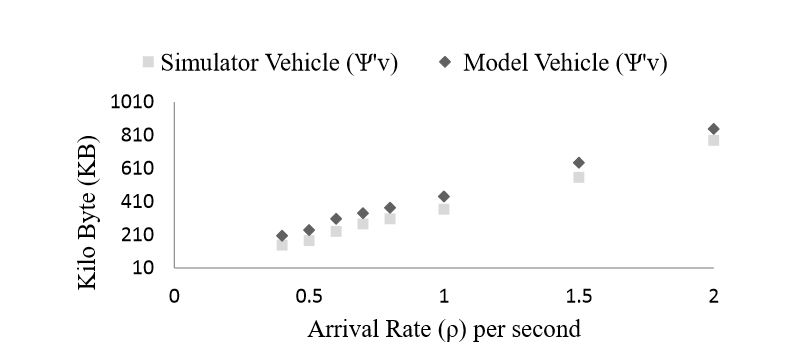
Figure 13. Comparison of the simulation and the model based vehicle storage requirements at different arrival rate
In the Figure 13, we compare the storage requirements of vehicles based on simulation results and the modeling results. In this case, we consider v = 16.67 m/s, L = 1000 m, rv = 200 m, ri = 500 m, na = 20 but ρ is varied. The simulation results are very close to the modeling results because both the model and simulation considers average number of platoon members. With the increase of arrival rate the storage requirement also grows.
DYNAMIC ADAPTATION STRATEGY OF THE SIOV
Characteristics analysis from the above section provides the basis for SIoV parameters relationships, which are further used to develop the adaptation strategies for individual physical component (i.e., subsystem) in this section. In SIoV every physical component is aware of its cyber entity by one-to-one mapping. As a result, any adaptation in the physical layer is notified to the cyber layer and vice versa. While designing the adaptation strategies, preferences are given to the locally known parameters over the global ones for every subsystem. Main objective of the adaptation techniques is to collect as much sensory data as possible while keeping the overall workload to an acceptable limit.
CONCLUSIONS AND FUTURE WORKS
Social Internet of Vehicles is a cloud based cyber-physical approach to tackle the internet of vehicles related issues in a more scalable and distributed manner. The cyber layer of the SIoV eases the integration of different IoT domains. Various relationship types and related policies open the opportunities of intelligent service compositions following social network rules. In this paper, we have designed the analytical models of the subsystems involved in the SIoV interaction process. The workload models for vehicle, infrastructure, home, and the cloud would help to understand the storage or computing requirements, which is vital for Big Data management.
Cloud computing cost can be balanced for SIoV related applications deployment using workload model based dynamic adaptation. In this paper, we provide detailed simulation results of subsystem workloads at different parameter settings. Based on the characteristics analysis of SIoV, we find the system parameter relationships, which are further used to design example adaptation strategies for different SIoV subsystems. The proposed models would be useful to deploy SIoV based safety, efficiency or comfort applications. Design and development of a robust cyber-physical IoV simulator that can incorporate adaptation strategies is our next future work.
Also, integrating real vehicles with the simulated vehicles under the IoV simulator platform can offer interesting insights about deployment experience. Integration of heterogeneous IoT domains through the cyber layer is another possible work. Since vehicular sensory data is a key element of sensing-as-a-service model hence we are working on the development a multimedia sensory dataset for the IoV applications. The dataset can include both OBD based vehicular data and Smartphone sensor readings.
Source: University of Ottawa
Authors: Kazi Masudul Alam | Mukesh Saini | Abdulmotaleb El Saddik
>> 200+ IoT Led Projects for Final Year Students
>> IoT based Networking Projects for Final Year Students
>> Simple Java Projects with Source Code Free Download and Documentation