ABSTRACT
In the blooming era of the Internet of Things (IoT), trust has been accepted as a vital factor for provisioning secure, reliable, seamless communications and services. However, a large number of challenges still remain unsolved due to the ambiguity of the concept of trust as well as the variety of divergent trust models in different contexts. In this research, we augment the trust concept, the trust definition and provide a general conceptual model in the context of the Social IoT (SIoT) environment by breaking down all attributes influencing trust.
Then, we propose a trust evaluation model called REK, comprised of the triad of trust indicators (TIs) Reputation, Experience and Knowledge. The REK model covers multi-dimensional aspects of trust by incorporating heterogeneous information from direct observation (as Knowledge TI), personal experiences (as Experience TI) to global opinions (as Reputation TI). The associated evaluation models for the three TIs are also proposed and provisioned. We then come up with an aggregation mechanism for deriving trust values as the final outcome of the REK evaluation model. We believe this article offers better understandings on trust as well as provides several prospective approaches for the trust evaluation in the SIoT environment.
AUGMENTATION OF TRUST CONCEPT IN THE SIOT
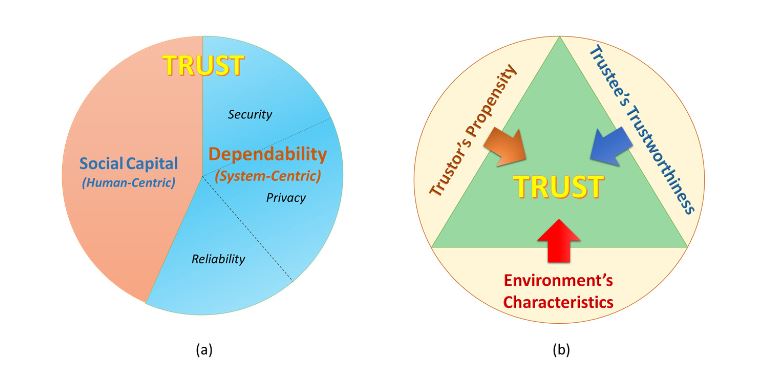
Figure 1. (a) Trust concept in the relation with dependability and social capital; (b) Three main aspects of trust in the SIoT environment
An advanced variant of trust for a computer system is dependability that is evolved from reliability, security and privacy considerations. Besides security and privacy, reliability is a factor showing whether a systems is going to perform properly. Thus, dependability is de facto property of a system representing ability of the system to deliver secure and quality services by characterizing the security, privacy and reliability schemes in terms of some attributes such as availability, safety, integrity, confidentiality and reliability.
Grandison and Sloman have defined this variant of trust as “infrastructure trust”. In our perspective, dependability is one of the most important indicator in evaluating trustee trustworthiness (in case the trustee is a computer system). The key distinction between trust and dependability is due to the enrollment of social interactions (of both humans and devices), which is modulated in form of social capital factors (Figure 1a).
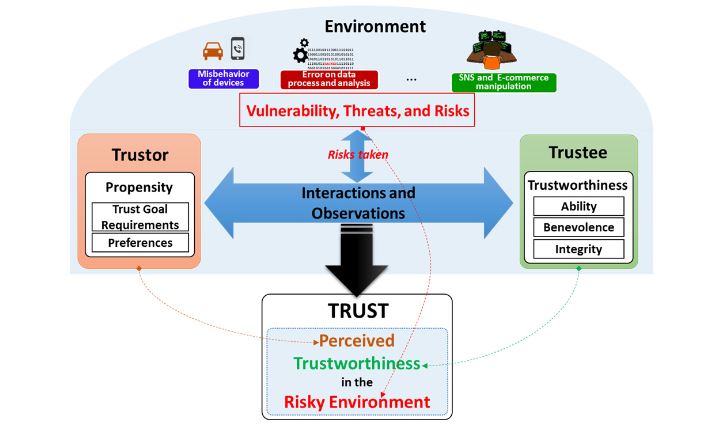
Figure 2. Conceptual Trust Model in the SIoT environment
According to the proposed model illustrated in Figure 2, trust will be obtained by harmonizing the trustor’s propensity and environment conditions into the trustee’s trustworthiness. The harmonization is accomplished by aggregating both the observation of a trustor toward a trustee and the interactions between the two. It is worth to note that the environment conditions are reflected as risks taken during the observations and interactions. The trustor’s propensity includes both requirements for the trust goal and the trustor’s preferences about the trustee’s trustworthiness whereas the environment conditions are the considerations for some factors such as vulnerabilities, threats and risks.
TRUST EVALUATION MODEL: BACKGROUND AND PROVISIONS
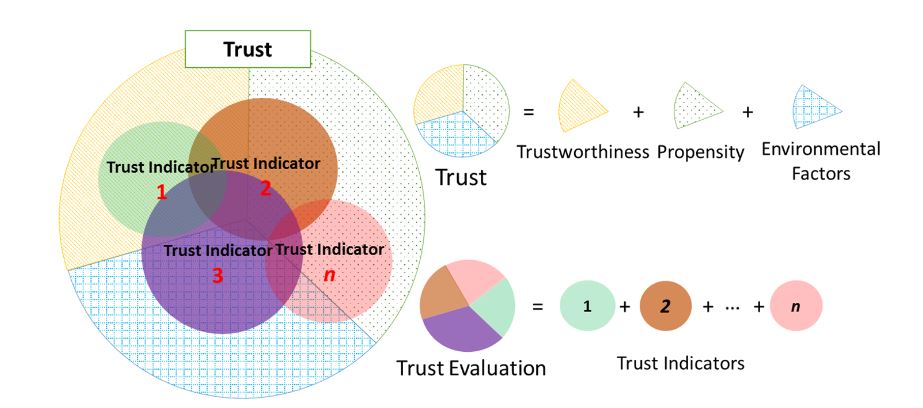
Figure 3. Concept of computational trust that comprised of multiple trust metrics
However, other TIs do not necessarily only stick to the direct trust evaluation scheme. Thanks to the integration of social networks, some TIs can be determined based on social interactions in the SIoT environment that effectively indicate trust such as Recommendation and Reputation which are evaluated contingent on the propagation characteristic of trust. These TIs are then combined to derive a portion of the complete trust called computational trust. The computational trust is persuasively used on behalf of the complete trust (Figure 3). As many TIs are specified and evaluated as more accurate the computational trust will get. However, as two sides of a coin, there is always trade-off between computational trust accuracy and computational efforts.
REK TRUST EVALUATION MODEL IN THE SIOT
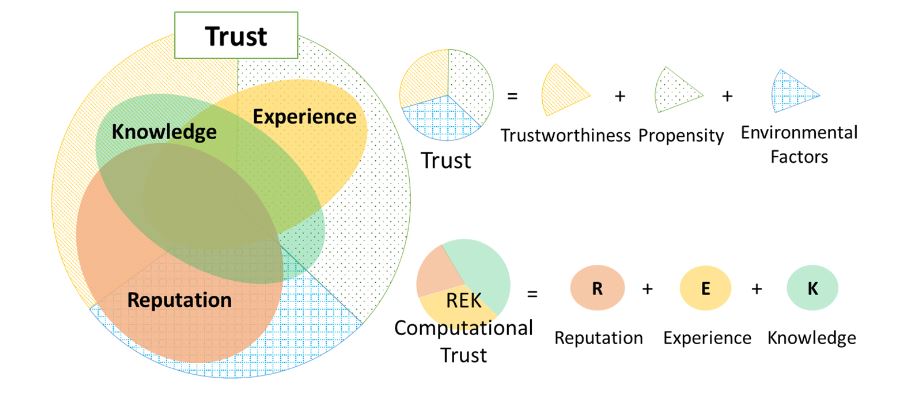
Figure 5. Reputation, experience and knowledge as the three indicators in the REK trust evaluation model
Knowledge TI is the direct trust mentioned in Section 3 that renders trustor’s perspective on trustee’s trustworthiness in a respective environment. Knowledge TI can be obtained based on limited available information about characteristics of the trustee and the environment under the trustor’s observation. Knowledge TI can reveal a portion of trust which is illustrated in Figure 5. It indicates more about trustworthiness of the trustee and trustor ’s propensity but not much about the environmental vulnerabilities, threats and risks.
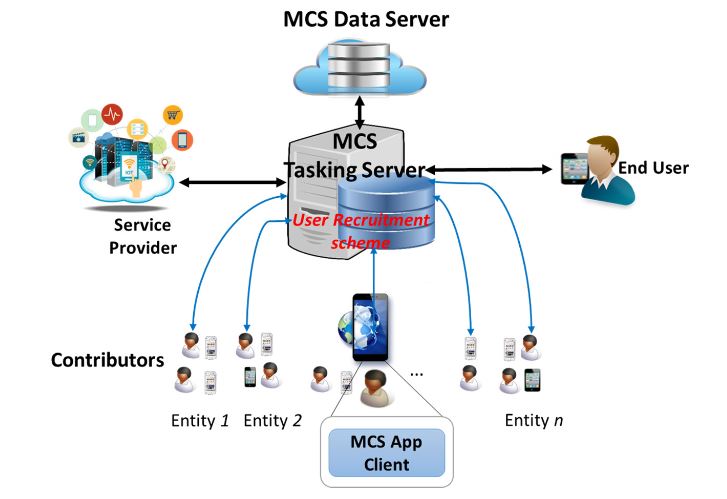
Figure 7. Mobile Crowd-Sensing System Architecture
One of the challenges in MCS is the recruitment of contributors for sensing tasks. In a crowded urban area with high number of participants, it is critical to recruit trustworthy users to collect high quality of data as well as to guarantee security, privacy and data integrity. This challenge calls for an efficient User Recruitment scheme implemented in the MCS Tasking Server for making proper selection of contributors respecting to a specific sensing task as illustrated in Figure 7 (the sensing task requested by service providers and assigned based on a mechanism deployed at the MCS). Note that in order to recruit users evolving in a sensing task, the MCS Tasking Server should manage an incentive scheme as rewards for their contributions.
CONCLUSIONS AND FUTURE WORK
In this article, we have provided a comprehensive understanding on trust concept in the SIoT with the REK evaluation model for trust which incorporates the three major TIs Reputation, Experience and Knowledge considering multi-dimensional trust aspects from direct observation to third-party information. We also have examined necessary TAs for covering the direct observation of trustworthiness as the Knowledge TI considering the three dimensions Ability, Benevolence and Integrity of any entities in the SIoT environment.
We have also proposed prototypes for the Experience and Reputation TIs by proposing the associated mathematical models leveraging the sociological behaviors of human in the real world as well as the Google PageRankTM ideas in the web page ranking areas, respectively. Finally, we combine the TAs of the Knowledge TI, the Experience TI and the Reputation TI using Semantic-Web technologies for finalizing the overall trust value as the level of trust. This article opens a large number of research directions in order to fulfill the trust evaluation platform. The first direction is to adapt the trust evaluation model to various scenarios and use-cases that require to figure out a set of TAs for Knowledge TI in detail as well as appropriate mathematical parameters for Experience and Reputation TIs.
The second direction could be a smart mechanism to reflect the trustor’s propensity and environmental factors to the trust evaluation model such as an autonomous weighted sum mechanism with machine learning for adaptively changes the weights according to a particular context. Another solution could be a smart rules generators for the trust knowledge-base so that the final trust value will be obtained in a context-awareness manner. In the demonstration in Section 4.5, the rules are predefined using understanding of a specific service with user preferences on trust. This can be improved by using machine learning techniques for rule pattern recognition in an automatic rule creation mechanism.
Source: Liverpool John Moores University
Authors: Nguyen Binh Truong | Hyunwoo Lee | Bob Askwith | Gyu Myoung Lee
>> 200+ IoT Led Projects for Final Year Students