ABSTRACT
Portfolios of financial instruments are designed to increase returns and manage risk. In high-risk investment strategies, central measures of risk must be complemented with controls on tail measures of risk. An unanticipated event that impacts securities of one firm can contagiously effect those of other firms through a contagion flow process that may occur via a set of network connections.
Such connections among firms arise due to a variety of factors, such as a shared supply chain member or auditing firm. These connections spread the contagion, potentially impacting numerous other firms in the network. This can adversely affect the level of tail risk in an investment strategy, especially when many such connected and affected firms are included in a portfolio, such as a bond portfolio. Reduced form models illustrate how connections between firms can lead to the heavy tailed default distributions seen in empirical data.
Historical events help in the understanding of connections that allow contagion to spread between firms. The goal of this research is to combine the insights gained from both previous models and historical events into a structural model for flow of contagion among firms. This includes defining and calibrating contagion variables, including the network that allows the contagion to spread between firms resulting in increased defaults for a portfolio of debt instruments.
The model is then utilized to assess the impact of the network structure on the portfolio of high-yield debt instruments. Additionally, simulation data from the model is a key input into a bond optimization used to provide guidance on the immunization of a portfolio of bonds from the impact of contagion flow. Two historical incidences of contagion are presented as test cases to validate the ability of the proposed firm value model including contagion, to alert investors to the impact of a contagion on a bond portfolio.
MODEL DEVELOPMENT: FIRM VALUE AND OPTIMIZATION
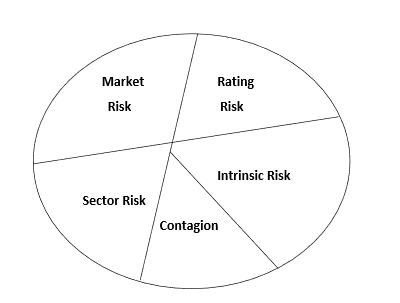
Figure 2.1: Disaggregated Risk Factors Impacting Firm Value
In a similar manner, this thesis models the return on firm-asset value by decomposing the total risk into four non-contagion factors where each has a unique role to play in determining the return on asset (ROA) in excess of the risk free rate. As depicted in Figure 2.1, a contagion risk factor is also included in the model as a fifth factor. Premiums associated with each of these risk factors determine the expected return on the firm’s assets.
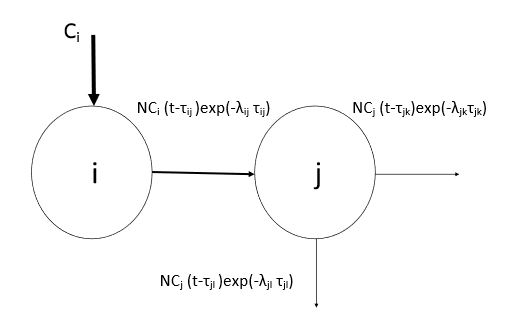
Figure 2.2: Firms i and j connected in a network
A firm can be connected to multiple other firms with the direction determined by the nature of the connection. As illustrated in Figure 2.2, firm i is impacted by an exogenous contagion. Firm i affects firm j, which in turn spreads contagion to two other firms, as illustrated by the outgoing arrows. Connections can be represented as basic structures.
CALIBRATION AND SIMULATION
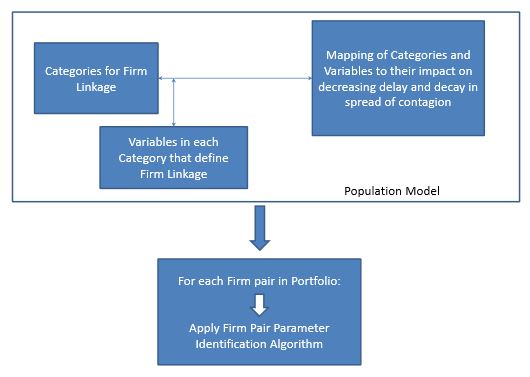
Figure 3.1: Framework to establish Contagion Spread Variables
The final procedure applies the output of the first three procedures to a set of firms being analyzed for inclusion in a portfolio. Information on firms is collected based on the first two procedures and combined with the constructs of the third procedure to assign a network structure for the firms considered. The layout of the framework is presented in Figure 3.1.
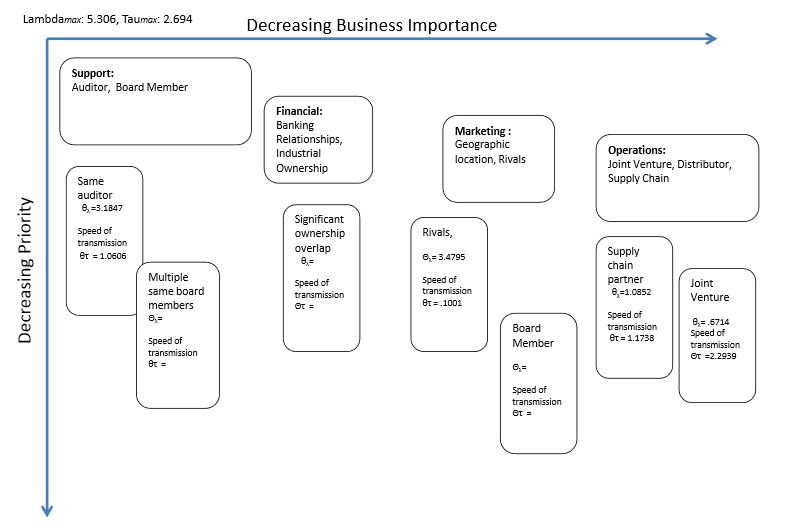
Figure 3.3: Connection strength by Category/Variable based on historical incidents
Researching firm information on Compustat and 10K reports revealed various connections as illustrated in Figure 3.4. BIIB and PDLI are connected by a joint venture. GILD and PDLI are connected by the use of the same auditor. All three firms are connected based on same Industry/Rivals. The connection strength for Industry/Rivals, however, wouldn’t be the same since BIIB and PDLI develop similar types of treatments (cancer and autoimmune) versus GILD (HIV and hepatitis).
CONTAGION-BASED FIRM VALUE AND PORTFOLIO SELECTION
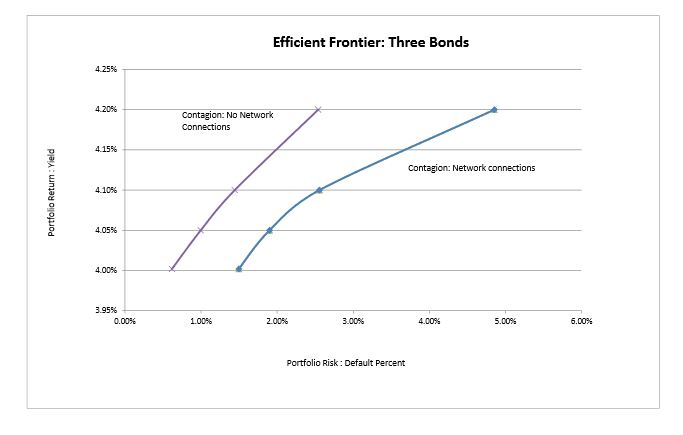
Figure 4.1: Efficient Frontier Three Bond Portfolio
Risk is determined by firm defaults since this is the risk factor most seriously impacted by a contagion. Additionally, the measure of portfolio risk, as described by equations 2.18, will be controlled to remain below a prescribed value. Figure 4.1 illustrates the contagion impact on the efficient frontier. The curve shifts to the right, showing an increase in risk for a given level of return when a network that allows contagion to flow is embedded in a portfolio optimization.
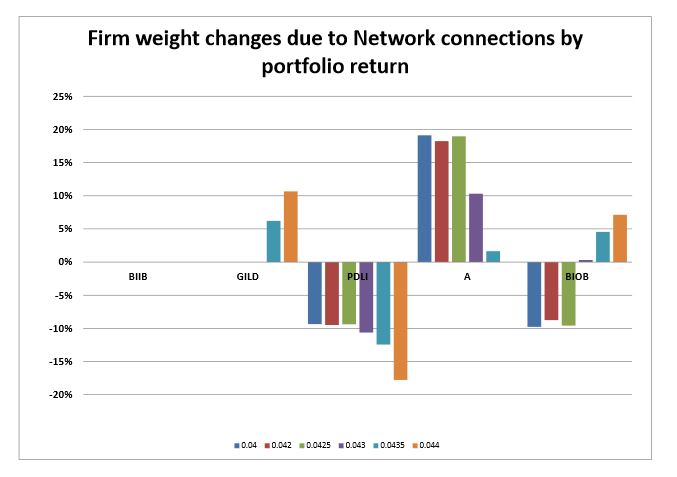
Figure 4.3: Portfolio Weight Changes due to Network Connections
The same impact due to contagion and the network structure is seen on the efficient frontier curve. Additionally, the composition of the portfolio changed, see Figure 4.3. With additional bonds choices (lacking any firm-pair connections), the weights of the firm-pair with the strongest connection stayed the same or decreased.
CASE STUDY AND COMPUTATIONAL ANALYSIS
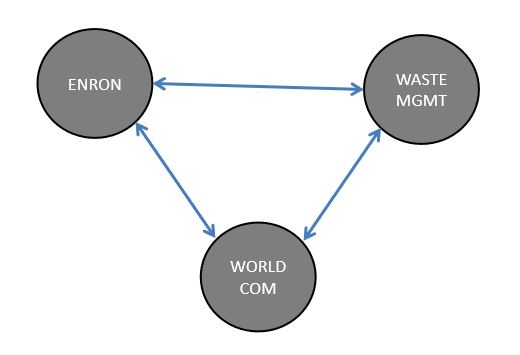
Figure 5.1: Auditor Induced Contagion
Historical data for the firm value model simulation will cover the period July 1, 1997-June 29, 2001. The contagion structure represents the spread of an exogenous contagion from any of the three firms to the other two firms, see Figure 5.1. Firm values are evolved bi-monthly over a one year period.
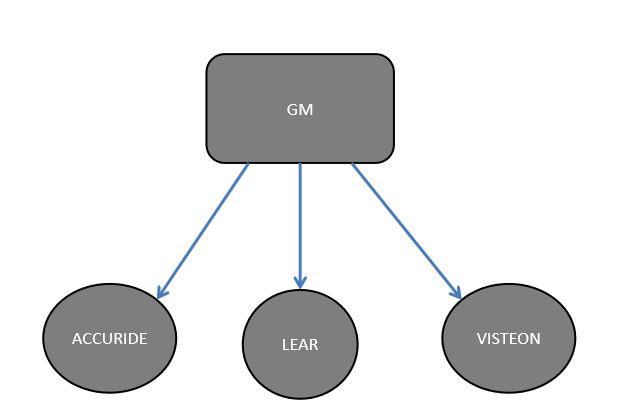
Figure 5.4: Supplier Induced Contagion
To illustrate the impact of problems at GM spreading to its supply partners, the firm value simulation was run based on historical data from 4th quarter 2002 to 4th quarter 2006. The structure has GM connecting to all three of its suppliers, see Figure 5.4. Firm values are evolved bi-monthly over a one year period. Contagion arrival is modeled as a Poisson process with an average of one arrival per year.
CONCLUDING DISCUSSION
Much research has been done to improve understanding of the causes of increased clustering in defaults, which is of particular concern in portfolio selection. Often the research focuses on contagion. Most models, however, lack the use of actual firm data and therefore the ability to increase the understanding of how the contagion process works between firms. The aim of this thesis is to develop a model that assists in the understanding of the impact of a network structure among firms on the spread of a contagion.
A structural model is developed to evolve firm value impacted by contagion in addition to commonly considered risk factors. Contagion, modeled as an exogenous event spreading from one firm to another due to a network structure, is segmented into variables increasing understanding of what characteristics impact defaults. Results from this model are then combined with other firm data to create a portfolio optimization with the goal of reducing exposure to multiple defaults.
Our research bridges the gap between stylized contagion models and the empirical data which illustrates the impact of contagion. Building on previous research, such as Egloffet al. (2007), a framework is developed to address the issue of calibrating the contagion pieces of the model through the use of firm qualitative data and historical contagion events. Thus developing a platform to analyze the impact of contagion when creating a portfolio of bonds.
Source: University of Tennessee
Author: Wendy Ann-Swenson Roth