ABSTRACT:
Ubiquity of mobile devices with rich sensory capabilities has given rise to the mobile crowd-sensing (MCS) concept, in which a central authority (the platform) and its participants (mobile users) work collaboratively to acquire sensory data over a wide geographic area.
Recent research in MCS highlights the following facts: 1) a utility metric can be defined for both the platform and the users, quantifying the value received by either side; 2) incentivizing the users to participate is a non-trivial challenge; 3) correctness and truthfulness of the acquired data must be verified, because the users might provide incorrect or inaccurate data, whether due to malicious intent or malfunctioning devices; and 4) an intricate relationship exists among platform utility, user utility, user reputation, and data trustworthiness, suggesting a co-quantification of these inter-related metrics.
In this paper, we study two existing approaches that quantify crowd-sensed data trustworthiness, based on statistical and vote-based user reputation scores. We introduce a new metric-collaborative reputation scores-to expand this definition. Our simulation results show that collaborative reputation scores can provide an effective alternative to the previously proposed metrics and are able to extend crowd sensing to applications that are driven by a centralized as well as decentralized control.
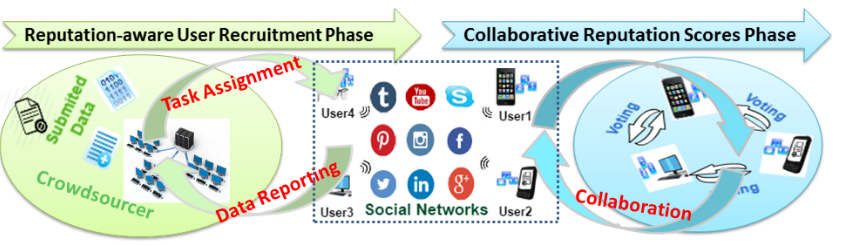
Proposed system model. The crowdsourcer (platform) adapts reputation-aware recruitment methods and assigns its participants (users) their sensing tasks.
SNIPPETS:
Background and Related Work:
A. MCS Applications:
More than 1.4 B smart phones and 232 M wearable appliances were sold in 2015, while the sales of wearable devices is projected to reach 322 M in 2017. Various phenomena such as air pollution, water quality, road conditions for smart transportation, public safety, and reparedness can be collaboratively sensed through these devices, or environmental monitoring can be performed through autonomous field systems MCS has attracted the IT industry for various applications.
B. Components of Mobile Crowd-sensing (MCS):
- User Recruitment
- Platform Utility And User Utility
- True Payments And False Payments
C. Factors Compromising User Participation in MCS:
Despite the rapid growth in the popularity of mobile devices, user participation in MCS is still lackluster due to the extensive consumption of time, energy, and bandwidth resources that a typical MCS application requires.
D. Participant Selection in MSC:
From the platform’s standpoint, a key consideration is the selection of the participants to maximize platform utility. A limited number of studies investigate different approaches to address user involvement in MCS to maximize platform utility.
Reputation and Trustworthiness in MCS:
In this section, we study reputation scores in two categories: i) statistical reputation scores that are solely computed by the platform and ii) vote-based reputation scores that are computed by the participants of the MCS.
In this subsection, we provide a high level definition of the two key terms of our study, namely user reputation and data trustworthiness. We associate the reputation attribute with a mobile user, while the trustworthiness is an attribute that is associated with the data a given user collects; hence, based on these definitions, the data trustworthiness is a direct consequence of user reputation.
PERFORMANCE EVALUATION:
In this section, we evaluate the performance of the proposed user recruitment schemes in MCS systems via simulations. We aim to quantify the user reputation and data trustworthiness under statistical and collaborative methods for user recruitment in MCS.
Furthermore, we investigate the impact of deploying anchor nodes as the trusted entities to improve the reputation of the recruited user sets. In our simulated scenarios, users are recruited based on collaborative reputation scores with/without anchor nodes (i.e., trusted entities) and TSCM.
CONCLUSIONS:
The emergence of cloud computing and Internet of Things (IoT) enables Mobile Crowd-Sensing (MCS) platforms to be formed, in which a community of mobile users use the built-in sensors in their mobile devices—such as gyroscopes, accelerometers, barometers, microphones, cameras, and temperature sensors—to sense several phenomena.
One for profit MCS application, Sensing-as-a-Service (S2 aaS), is a promising recipe for the commercialization of the MCS-acquired data by compensating the participants of the MCS community monetarily and selling the data to interested parties. Alternatively, many not-for-profit applications exist that formulate the usage of the MCS-acquired data for environmental monitoring and other community enhancement initiatives.
In this paper, we present a detailed MCS performance study, centered around these three metrics under multiple user recruitment policies. All of the schemes adopt a reverse auction-based user recruitment procedure but differ in their trustworthiness assurance methods. Among these, TSCM runs a statistical reputation-based method, which keeps track of true and false readings through outlier detection techniques.
Our simulation results highlight the following results:
- Using collaborative reputation scores in user recruitment improves platform utility and data trustworthiness by reducing false payments (rewards to malicious users).
- Deployment of anchor nodes to assist in the decentralized (i.e., vote-based) component of collaborative reputation scores helps in reducing the false payments, however there is no clearly-evident advantage to use anchor nodes when user reputation scores are collaboratively calculated. Previous studies show an improved platform utility under certain circumstances when the user reputation scores are calculated in a fully decentralized fashion.
- When collaborative methods are employed, using statistical reputation in the assessment of the value of a recruited crowd can reduce the user bias in the decentralized vote-based component of the reputation score; consequently, it can help reduce the negative impact on user utility.
- Our evaluation of the initial reputation of the newly joining users shows that setting the initial reputation to a value of ≈0.3 leads to the most feasible results in terms of platform utility, user utility and data trustworthiness.
We are currently working on incorporating mobility models as well as inter-participant interaction in an MCS platform. As the aim of this paper is laying the foundations of quantifying user reputation in crowdsensing systems, performance study has been limited to simulation results. However, we are currently working on integrating the methods that are presented here with a small-scale crowdsensing testbed to run tests with real multi dimensional data.
Source: Clarkson University
Authors: Maryam Pouryazdan | Burak Kantarc | Olga Soyata | Luca Foschini | Houbing Song
>> IoT based Big Data and Cloud Computing Projects for B.E/B.Tech Students
>> 200+ IoT Led Projects for Final Year Students